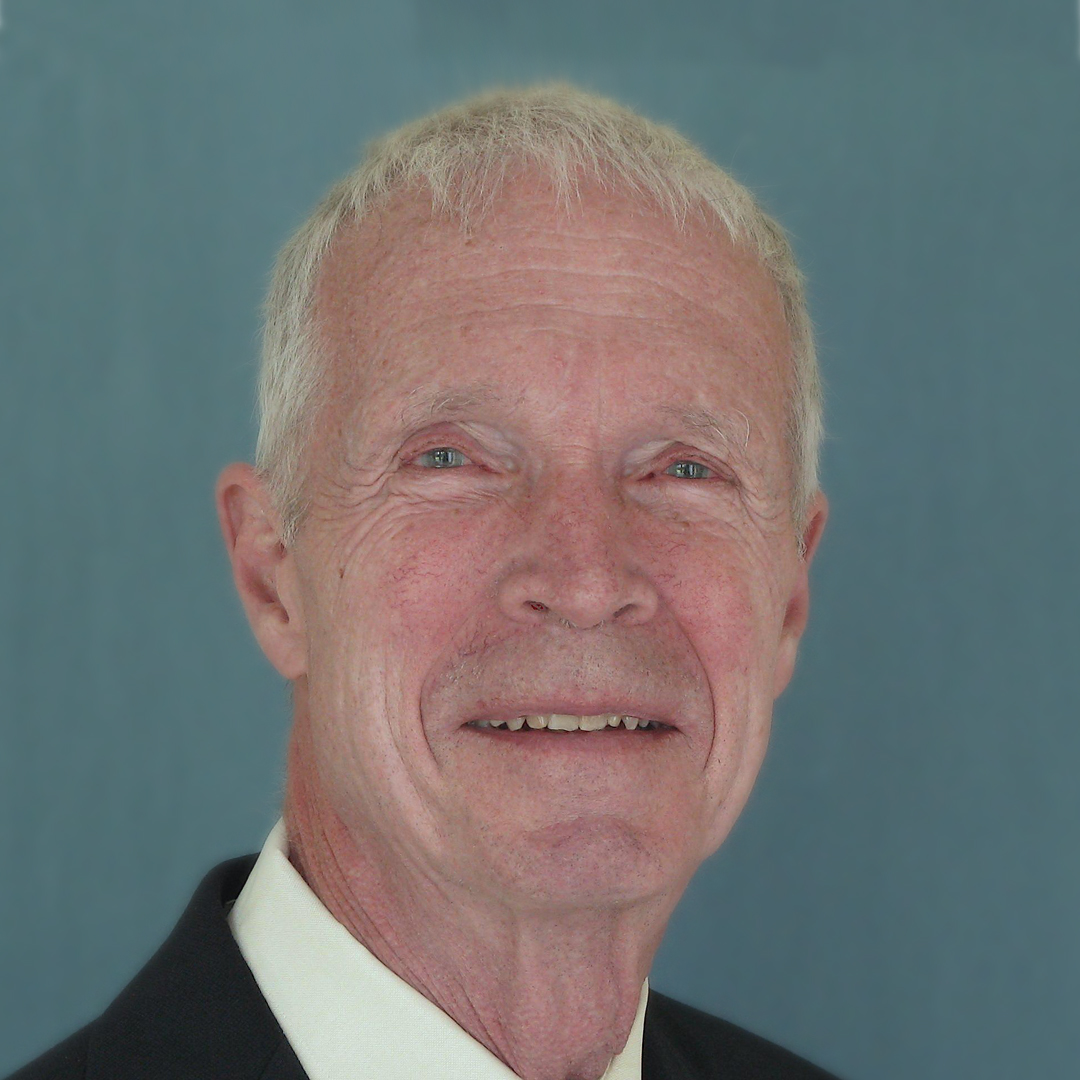
It’s official. Our technology has begun to learn from the world around it. As you read this, someone’s smartphone is teaching itself to recognize its owner’s face. A self-driving car is learning to drive by watching us behind the wheel. The origin of this “machine learning” revolution can be traced back to John Hopfield, whose career is as fascinating as the technologies his ideas helped foster. Hopfield earned his Ph.D. in physics but is now a professor of molecular biology at Princeton. Over time, his research meandered from hard physics to neuroscience, where he applied his knowhow from the former to construct an artificial neural network capable of modeling certain functions of the human brain. Just as hearing a song can bring back the memory of an old friend, Hopfield’s neural network allowed machines to store simple memories and, like our brains, recall them with only partial information. Decades later, these fundamental concepts have helped to unleash the tide of “deep learning” technologies that allow machines to observe, remember, and learn on their own.
John Hopfield, professor of molecular biology at Princeton University, began his career as a solid-state physicist. Over nearly six decades, he has used his knowledge and experience to cross borders between scientific disciplines, for example, to illuminate physical concepts underlying biological and biochemical processes. A true adventurer, Hopfield is not focused on disciplinary constraints; he cares more about asking questions, tackling potential solutions, and moving on once the problem yields.
Born to two physicists, as a child, Hopfield built model airplanes and crystal set radios. He enjoyed blanket permission to dismantle whatever he wished in the house—as long as everything was returned to its original condition. Becoming a scientist or engineer was never a question: Hopfield’s primary motivation had always been to understand how things work. Whether pertaining to cosmology, biology, the transistor, or the human brain, the physics of our world has been a constant inspiration.
Hopfield earned his undergraduate degree in physics from Swarthmore College in 1954 and completed his Ph.D. at Cornell University in 1958. His career as a solid-state physicist (using quantum mechanics, crystallography, electromagnetism, and metallurgy to study solid matter) began in earnest in 1958 after he joined the prestigious Bell Laboratories as a member of the technical staff. He attributes his tireless work ethic to the experts he encountered at Bell Labs and the quality of their research, and to the high standards he had to meet there.
While at Bell Labs, Hopfield learned that his talent was often best expressed in finding simple answers to complex problems. But the more he learned about a topic, the deeper his questions probed and the more difficult it became to limit his inquiries to the field of solid state physics. His scientific contributions began to intersect with many fields, and would inspire not only fellow physicists, but, also biologists, engineers, computer scientists, and psychologists.
A major contribution to the field of genetics came in 1974, when Hopfield demonstrated that the high degree of accuracy present in genetic expression could be explained by coupled chemical reactions called “kinetic proofreading.” Hopfield described kinetic proofreading as a mechanism for error correction in biological reactions, like such as protein synthesis. It is essential in all steps of gene expression and in the immune system’s ability to identify foreign substances.
But Hopfield was not one to linger on any particular question for too long. He describes himself as the type of person who will try most anything but prefers to move on once his understanding reaches an acceptable level. It is why, he says, he is a poor piano player, sailor, skier, tennis player, and golfer—but it also provokes him to ask more complex questions of the world around him. In the 1980s, Hopfield boldly focused his attentions to the most complex and elusive system in the Universe: the human brain—whose mysteries he continues to pursue today, nearly 40 years later.
In 1982, Hopfield developed a model of neural networks to explain how memories are recalled by the brain. The Hopfield model explains how systems of neurons interact to produce stable memories and, further, how neuronal systems apply simple processes to complete whole memories based on partial information. This neural network approach to memory further emboldened a new generation of physicists to look with fresh eyes at other complex interacting systems and branch out into other areas of science.
The contemporary impact of the Hopfield model is evident in fields as diverse as physics, biology, and computer science. By constructing an artificial neural network capable of modeling certain functions of the human brain, machines can now use these processes to store “memories.” This technological advance unleashed a tide of deep learning technologies in which machines learn from the external world: Smartphones recognize our faces or fingerprints to unlock. Self-driving cars learn to drive by watching us behind the wheel. Our machines now observe, learn, and remember on their own. Hopfield’s work expanded the horizons of physics, making it clear how local computations could give rise to global outcomes.
The scope of Hopfield’s many awards reflects the interdisciplinary nature of his career. His honors include the Society for Neuroscience Prize for Theoretical and Computational Neuroscience (2012), the Harold Pender Award from the University of Pennsylvania School of Engineering and Applied Science (2002), the Dirac Medal and Prize from the International Center for Theoretical Physics (2001), and the International Neural Network Society’s Helmholtz Award (1999).
Information as of March 2019